Fluorescence Lifetime Imaging (FLIM) is an attractive microscopy method in the life sciences, yielding information on the sample otherwise unavailable through intensity-based techniques. FLIM microscopy images the environment and interactions of specific dyes and proteins in living cells. FLIM is also increasingly used to map viscosity, pH, ion concentrations and temperature in living cells, and efforts are underway to use FLIM for drug discovery and FLIM of auto-fluorescence for clinical diagnostics.
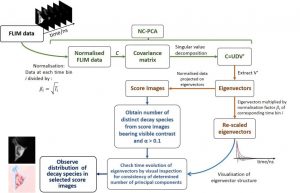
Workflow of the NC-PCA algorithm applied to FLIM data. The section in green represents the PCA procedure accounting for Poisson detection noise (NC-PCA). The section in orange represents the extraction of NC-PCA results. The section in blue represents analysis of FLIM data based on NC-PCA results.
In addition to FLIM probe and detector development or its combination with other techniques FLIM data analysis is an important aspect of ongoing progress in the field. The underlying assumption in FLIM data analysis is often that separate emitting species, states or microenvironments exist, which lead to distinct fluorescence decay profiles under different biophysical conditions. The decay in each pixel can then be modeled by a linear sum of exponential decay components. These decay curves can be fitted and various fitting procedures allow quantitative estimation of the decay parameters (lifetimes and their respective amplitudes). However, pixel-wise fitting models usually require prior knowledge of the photophysics of the probe in the conditions of interest, which is not always available or easy to obtain.
German and British researchers tested a novel Noise-Corrected Principal Component Analysis (NC-PCA) method for time-domain FLIM data. PCA is a statistical method designed to project multivariate datasets onto a subspace of reduced dimensionality, calculated to preserve the maximal variability contained in the data yet optimally reducing the noise. PCA has been used in other fields but has never been applied to FLIM data, possibly due to the complications introduced by the Poisson noise of photon detection. The research shows that this approach, which takes into account the Poisson-distributed noise inherent to single-photon detection, accurately resolves the number of emitting components and their distribution without requiring prior knowledge of their individual decay profiles. This makes NC-PCA a useful novel technique for FLIM data processing, due to its robustness in unfavorable conditions such as low intensity, presence of non-fluorescent background and poor component separation.