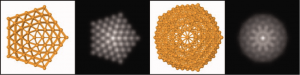
Structures and simulated STEM images for a 309-atom cluster: From left to right we have an ino-decahadron, a modeled STEM image of the same structure, followed by an Icosahedron also with its resepctive modeled STEM image.
The natural process of evolution, over the millennia, has led to the development of several unique genetic adaptations in organisms that maximize their survival in the ambient environment. A mathematical variant of this process of evolution (or natural selection) called genetic algorithm (GA), has been used over decades to solve very complex problems in diverse areas such as scheduling, optimization, and antenna design. The strength of many genetic algorithms lies in their mimicking of natural evolution processes that have been perfected by nature over a very long period of time.
Since the modern re-invention of scanning tunneling electron microscopy (STEM) by Crewe et al., this technique has been used for elemental identification over sample areas, among many other uses. Spherical aberration corrected STEM data exhibits near-atomic scale resolution, which makes 3D nanocluster identification possible. In a recent paper in the Journal of Computational Chemistry, Roy Johnston and his group at Birmingham have identified this correction as being somewhat complicated and demonstrated an alternative GA-based method.
Using icosahedral and decahedral structures to parametrize their GA process, they found that overall; random minimizing search process bests a GA process for the icosahedral structure, while the lower symmetry decahedral structure, the GA process produces faster results. This may be understood in terms of the higher complexity (lower symmetry) problem being more easily solved using GA methods. Given the complexity of most STEM data, it is clear that GA-based methods can be expected to a significant performance enhancement in terms of global minimum search.
In retrospect, this should not be surprising. GA, and the process of evolution that it attempts to mimic, works well in really complex environments. Which is just fine for those of us looking to find nanoclusters in complicated STEM data.