Machine learning grabbed the headlines a few years ago when AlphaGo was able to defeat the reigning “Go” world champion. Since then developments have been gathering pace, with the technology finding a wide array of uses, from chemistry to facial recognition, and entertainment.
In Holland, a team of researchers have demonstrated the utility of machine learning in metamaterial design. Metamaterials’ properties come not as a result of the material’s chemistry, but rather, their shape and structure.
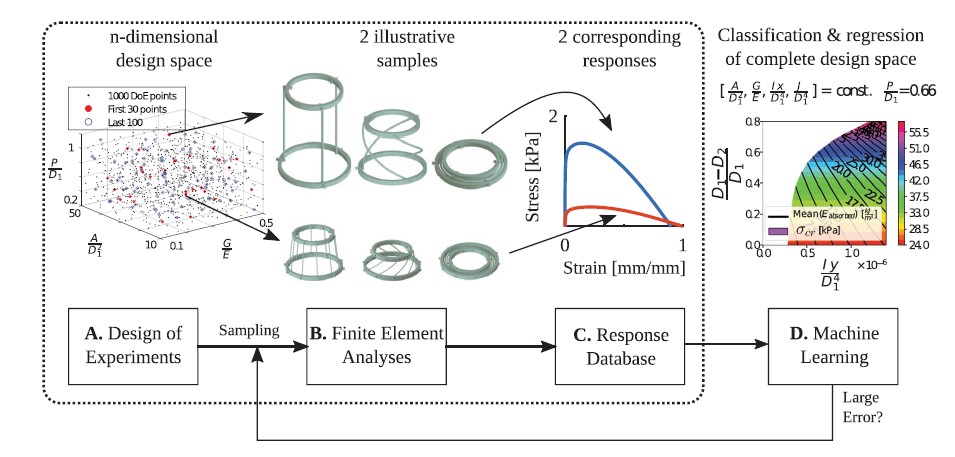
The team ran simulations of randomly generated designs whose geometry was defined according to seven separate variables. The effective compressive strains and stresses of the material were then predicted. This process generated 100 000 simulations. Once this process was complete, the algorithm analyzed the results and decided which section of design space should be further sampled (see figure above). The code for this process is freely available and can be found here.
The utility of the program was demonstrated by testing it on a cheap material that can be readily used in hobbyist 3D-printing devices. This printing technique tends to produce parts with defects, which can lead to brittle designs. These constraints were taken into account when choosing which type of design should be analyzed by the machine learning program.
Based on the results of the first classification and modelling step, the team selected six designs to print. The image below shows the design with the highest compressibility. This design was then selected to be printed at nano-scale. Interestingly, this process showed some of the limitations of the machine learning process.
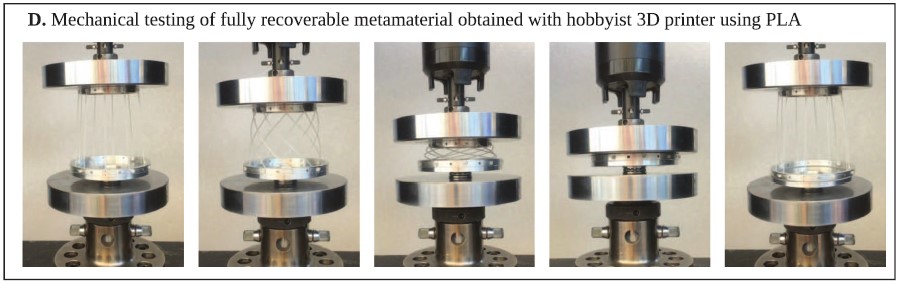
The photo-resist resin that the team used for nanofabrication shrinks significantly on curing. This caused defects in the resulting designs. The team were able to use the design charts produced by the simulations to tweak their design, and the resulting device, imaged by scanning electron microscopy, is shown below.
As shown by the difficulty with nano-fabrication, machine learning is only as good as the data that is supplied to it. But if the data is well curated, and the source of any errors well understood, then the results can be quite impressive. With the code and the manuscript freely available for use by anybody, the applications for this new approach to design are limitless.