Why do we need to discover new materials? The motivation for discovery is often to fulfill a societal need through the improvement of the performance of a system, process or product that exists or the development of one that never existed before. Incentives for materials discovery usually relate to real world issues, including manufacturing and business requirements, science policy and priorities, public policy and urgencies, national and international security and politics.
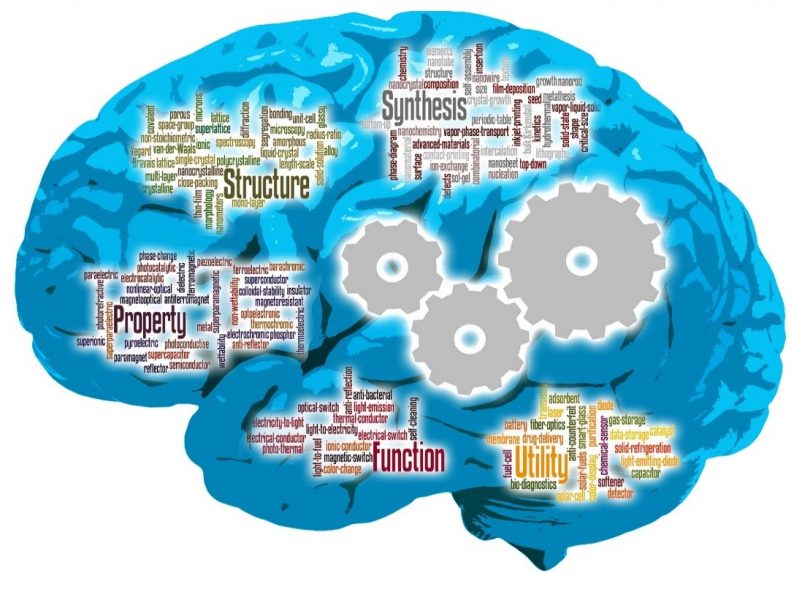
Figure 1. How do synthetic chemists and artificial intelligence learning machines think about materials discovery?
As a practitioner and observer of materials chemistry for half a century, I would argue that the traditional chemistry approach to materials discovery begins with synthesis, follows with connection of structure to properties, continues with associations of properties to function and culminates with utility and visions of new technology, Figure 1. Climbing these steps of the materials discovery ladder requires embracing a vast body of experiential knowledge accumulated over years of first-hand experimental and theoretical learning. Can a machine match or supersede a human in this task?
Today, I would argue, we have reached a point in the evolution of materials chemistry where the discovery of a new material or novel material property will be a rare event, especially in comparison to the past fifty golden years of materials discovery. By this, I mean that essentially every combination of elements from the periodic table has been explored in either a serial or a parallel fashion, with their constituents juxtaposed in the geometries of all known structures to tailor specific properties, evoke desired functionalities, and inspire diverse applications.
To be clear, we are not suggesting, “Everything that can be invented has been invented.”
There will of course always be the occasional eureka, most likely experienced with decreasing frequency than in the past, exemplified by the topological insulator, silicon laser, photonic bandgap, metamaterial, organic superconductor, polymer laser. Their novel properties will evoke new functionality, which will drive innovative technologies that will change our world.
One could make the case that we simply do not need any more new materials. Nevertheless, this should not stop us from trying to do so — following the lead of nature, which, presumably, has experimented with nearly every form of creative process over the past 4.54 billion years for the Earth and 13.8 billion years for the Universe.
To amplify upon the above this statement, we currently have easy access to massive libraries of materials and their elemental compositions, structures (be they crystalline, quasi-crystalline or amorphous), morphologies, stoichiometry’s, defect populations and length scales. These qualities can deliver essentially any material property or function required for almost any imaginable application. A difficulty however, sometimes seems to be achieving a combination of properties and functions for a certain application, for example, inexpensive, highly active and non-toxic catalyst materials.
Therefore, one could claim the way forward is not to only promulgate the incessant search for new materials by using either human or artificial intelligence. Instead, or rather in conjunction with this search, we should learn how this cornucopia of known materials, with their different sizes, shapes and surfaces, could be assimilated into a new generation of self-assembled material architectures, with form, function and utility intentionally designed to address modern societal needs. This should be one imperative and trajectory of materials science and materials engineering: assembling known materials in novel ways using new means, too.
If this provocative statement is true, then the entire field of “materials discovery” will be covering its bases, so to speak, exploring these two complementary approaches — both of which can focus on the possibilities of materials engineering and processes, devices and products derived from the engineering of existing materials and perhaps, new materials as well.
This dual approach to creating and discovering a treasure trove of next-generation materials considers existing libraries of materials and nanomaterials as “modular construction units” or “building blocks”, to be organized into higher-level architectures over multiple length scales through different modes of self-assembly: co-assembly, directed assembly and hierarchical assembly. Unlike those of traditional materials assembly processes, the driving forces of self-assembly can operate over length scales from the nanoscale (short-range) to the macroscale (long-range) interactions.
Both approaches will surely require machine-enhanced human creativity. Moreover, both would open up the whole field of materials rather than shutting down a once productive line of discovery as if it were selected for extinction by some evolutionary process.
Further building on this point and weighing in on the possible contributions of AI & machine learning: It may be premature to rule out the possibility that the next generation of creative thinking machines could design and engineer new materials by combining all five assembly processes in purposeful ways nanochemists and materials chemists haven’t fully anticipated or considered to date. Leveraging the advent of AI systems, we may be able to simultaneously pursue both paths: the unknown one to new materials discovery and the known one to combining and constructing existing materials. That said, it may be premature to rule out the possibility that the next generation of thinking machines could design and engineer new materials by combining all five assembly processes in purposeful ways nanochemists and materials chemists may not have anticipated or considered to date
The synthesis and assembly of these architectures is not restricted to chemical forces, be they covalent, electrostatic, hydrogen bonding, dipole or Van der Waals, but also include physical forces such as capillary, colloidal, electrical, magnetic, optical, elastic, convective and shear.
In this context, one can think of self-assembly traversing all size scales as “panoscopic”, with five guiding principles concerning the properties, interactions, dynamics and organization of the building blocks, as summarized below:
(i) Structure and properties – composition, doping, defects, size and shape, surface structure, charge, functionality, degree of perfection.
(ii) Attractive and repulsive forces – energy minimization, equilibrium separation, colloidal stability in solution.
(iii) Reversible association and dissociation – assembly and disassembly, adaptable motion and corrective movements, lowest energy structure with minimal defects.
(iv) Interfaces and interactions – gas-liquid, liquid-liquid, solid-liquid, solid-solid, templates, surface patterns.
(v) Dynamics – Brownian motion, agitation, chemical and physical propulsion, dynamic assembly.
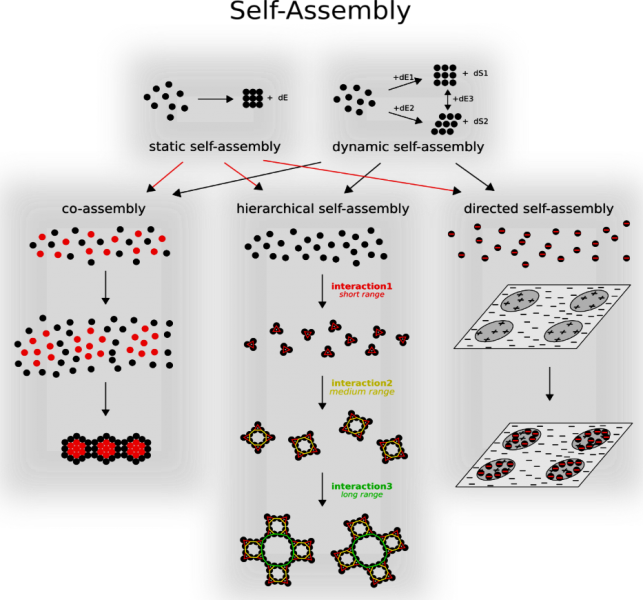
Figure 2. Self-assembly of modular building units over “all” length scales. Ludovico Cademartiri and Geoffrey Ozin, Concepts in Nanochemistry, Wiley-VCH, 2009.
To expand upon these five guidelines, Figure 2 depicts the major classes of self-assembly. The boundaries between them are explicitly blurred to emphasize the classification is inevitably imperfect.
Two main families of self-assembly exist, static and dynamic. These families both sub-divide into co-assembly, hierarchical assembly and directed assembly. The three sub-families are also interdependent and materials obtained via combined processes, such as hierarchical co-assembly or hierarchical directed self-assembly.
Co-assembly is a self-assembly process wherein two or more components contemporaneously self-assemble to form an architecture in which the initial components may be intermixed or segregated.
Hierarchical self-assembly occurs when the fundamental building blocks, self-assemble into primary structures held together by short-range forces. Such primary structures become the building block for the self-assembly of secondary structures, which occurs due to different, medium-range forces. This process can further repeat itself driven by longer-range forces until the highest level of self-assembly is attained.
Directed self-assembly occurs when an intentionally created and controlled external force is used to influence, or direct, the self-assembly of the fundamental building blocks.
Different varieties of self-assembly are often indicated by the forces involved therein. For instance, a patterned surface with islands of positive surface charge in a sea of negative charge can enable directed self-assembly of negatively charged building blocks to the positive areas in a process dubbed electrostatic self-assembly.
Porous materials with voids of different dimensions and shape can also direct the assembly of building blocks occurring therein, form a composite architecture in a process referred to as host-guest inclusion. This is an example of template-directed self-assembly.
This strategy for synthesizing panoscopic materials, assembled from modular building units over all length scales, from the nanoscale up to the macroscale, is enabled by a kind of human intelligence that differs from that used in making traditional materials.
I propose that we have reached a point in the development of materials chemistry where we have discovered a large fraction of the necessary building blocks in the materials construction set. The task, as I see it, is now a materials engineering challenge of finding ways to “build the house from the bricks”, to invent functional and purposeful constructions, rather than focusing efforts on discovering new bricks.
The future will tell whether artificial intelligence, trained to accomplish tasks as well as continual learning, intuition, and creativity, can match or supersede human intelligence in understanding, controlling and applying the forces of self-assembly to create functional constructs for use in advanced materials and biomedical science.
However, what if the most sentient technology is modeled, built and applied differently than the current machine learning systems today that deploy artificial intelligence. Imagine when thinking machines are designed to be more intuitive and creative—providing novel thought processes we have not yet characterized and categorized much less understand?
Maybe, this broader question needs to be explored: How could better designed, artificial intelligent systems connect-discover-invent-apply information in ways that enable them to combine the best practices in innovation of materials chemists and materials engineers, among other researchers in pursuit of new materials?
The first and obvious challenge of designing such a machine intelligence for materials discovery lies in the very fundamental principles of materials construction. In terms of searching out materials for specific tasks and purposes, the development of such a computer system (including both hardware and software) might be not only a design problem for machine learning algorithms, but also a test bed for computational complexity theory. Among all the quantitative parameters for constructing a definite type of material with a specific function and utility, the defects and degree of imperfection are two important, yet poorly defined, indicators.
With the efforts of the best human scientists and computer programs, it should be possible to convert these indicators to variables (numbers) with many degrees of freedom, in a multi-dimensional space. Very likely, the search for a best material for a specific purpose would be at least an NP-hard problem where NP stands for a non-deterministic polynomial-time in computational complexity theory. To solve these problems, some human intervention would be necessary, such as making approximations, randomizations and restrictions.
It will be interesting to see if artificial intelligence and machine learning is capable of thinking not just like a materials chemist but also like a materials engineer in the discovery of hierarchical constructions of the kind described in this article and envisioned for next generation materials-enabled technologies.
Written by:
Geoffrey A Ozin1, Chenxi Qian2, Todd Siler3
1 Materials Chemistry Research Group, University of Toronto, Toronto, Canada, Email:[email protected], Websites: www.nanowizard.info, www.solarfuels.utoronto.ca, www.artnanoinnovations.com
2 California Institute of Technology, Pasadena, California, USA.