Engineers commonly use cellular materials to reduce weight without impacting strength or stiffness. For instance, honeycombs are a natural example of such structural lattices. The geometry of the building blocks determines the structural properties of these lattices. Recently, researchers have become particularly interested in the development of auxetic metamaterials. Auxetics are a special class of cellular materials that have a negative Poisson’s ratio — that is, the cells become wider perpendicular to the direction they are stretched. This seemingly simple behavior has a profound impact on how materials can be used in applications that require deformation. Because of their unique behavior, auxetic lattice materials are useful in biomedical applications, sportswear, and body armor, as they also tend to express excellent energy absorption and flexibility.
However, bulk engineering materials rarely possess this property, so researchers must identify cellular designs and processes that exhibit negative Poisson’s ratios through the use of geometry. Currently, engineers manually design unit cell geometries by iteratively altering the connectivity to tune behavior. One of the most common methods of manufacturing these cellular structures is by using additive manufacturing, also referred to as 3D-printing. During the design process and before fabrication, the cellular structures are simulated to see how close the design is to achieve the intended behavior. However, calculating the mechanical properties of many proposed structures requires long computation times, which limits methods to identify novel cellular geometries with negative Poisson’s ratios.
Now, researchers from UC Berkeley are utilizing deep learning to bypass iterative finite element simulations. Their deep learning model, which is adopted from computer vision and image processing techniques, is trained using previously generated simulation data to predict the deformation behavior of favorable designs.
“This work is really exciting because we use the same basic architecture of models as those used for image detection and facial recognition, but instead of predicting what images appear in a picture, we train the model to predict the deformation behavior of a unit cell geometry” said Charles Yang, one of the co-authors of the paper in which his team’s findings are reported in Advanced Engineering Materials.
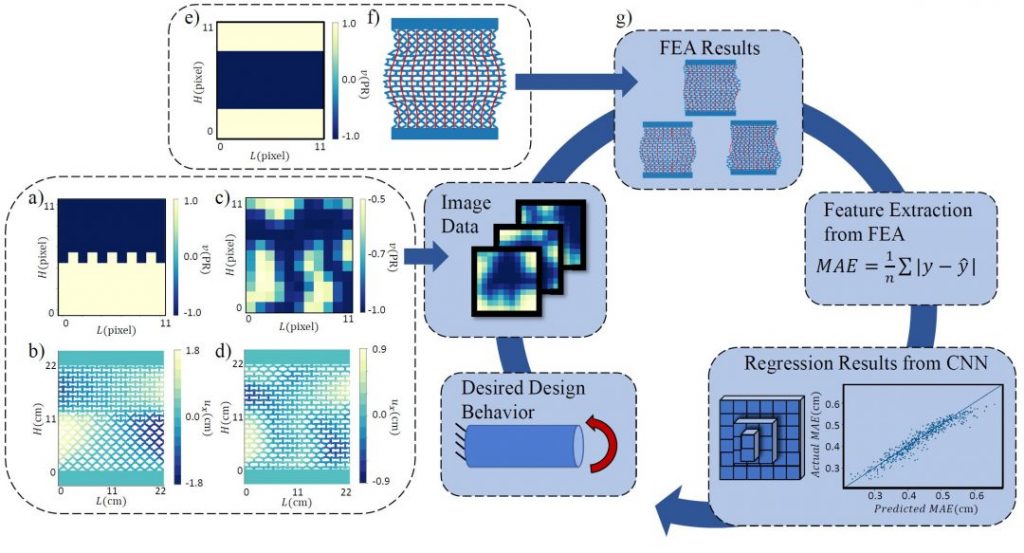
“After generating these complex lattices computationally, additive manufacturing is used to fabricate the designs, allowing for rapid prototyping and iteration,” said Professor Grace Gu from the Department of Mechanical Engineering at UC Berkley.
The machine learning workflow highlights design processes for future materials engineering. However, researchers emphasize the practicality of the application and ideal outcomes for future research. “A promising future work can involve exploring machine learning methods to develop new analytical models for auxetics which can be invaluable to understand the physical basis of material systems.“ said Jackson Wilt, who is the first author of the study.
Kindly contributed by Grace X. Gu.