Drugs are usually small organic molecules that bind to disease-causing proteins in order to neutralize their harmful effects. These proteins can be described at the atomic level, and the resulting information can be used to generate mathematical models able to guide the design of better drug candidates.
Until recently, these models employed classical linear regression, which limited their accuracy. As the sizes of relevant datasets grow, algorithms able to learn from them are achieving models of increased accuracy and broader applicability. Devising and applying such algorithms is the object of a research area known as “machine learning” (ML), the most successful subdivision of artificial intelligence. This approach is particularly timely given the amount of relevant data now available along with the maturity of ML algorithms.
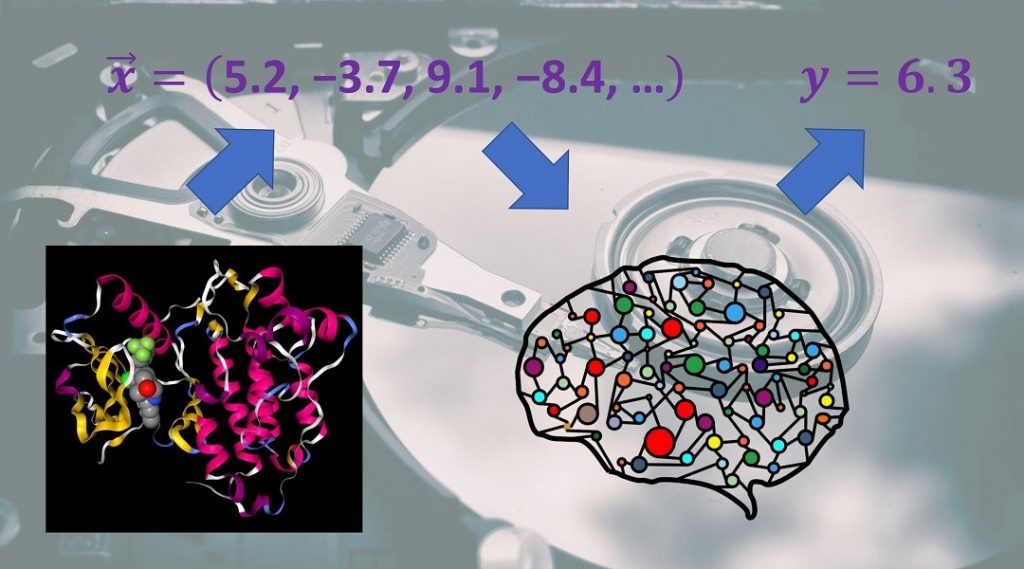
In a recent publication in WIREs Computational Molecular Science, Hongjian Li, Kam-Heung Sze, and Gang Lu from the Chinese University of Hong Kong, in collaboration with Pedro Ballester from INSERM in France, review the state of the art in ML research.
The performance gap between classical and ML-based models, which was already large, has now widened owing to further methodological improvements. This is also the case for target-specific models, although there are a few exceptions that might be due to having insufficient data for that target. Also, against the expectations of many experts, deep-ML algorithms have not always been more predictive than those based on more-established ML techniques.
Instead, the most successful strategies to generate predictive models have been those identifying better combinations of ML algorithms with numerical descriptions of the protein–drug interaction. There are also more of these models that are freely available for others to use, which is important for promoting their application to real-world problems.
For example, it is often the case that initial drug candidates do not bind sufficiently tightly to the protein and thus are unable to neutralize its effects completely.
A way to increase the potency of a candidate is to make and experimentally test a wide range of its chemical derivatives. Often, the number of derivatives that need to be considered is such that one cannot test all of them experimentally due to time and cost constraints. In this case, a predictive ML model would greatly shorten the time and cost of identifying potent drug candidates by only testing those derivatives predicted to bind most tightly to the protein.
Studies intended to elucidate which type of numerical description works better for a given target are expected to increase in the future. Another probable future trend will be to investigate which targets can be better modelled when their datasets are complemented with datasets from other targets — for example, by exploiting inter-target similarities.
Kindly contributed by the authors
Research article found at: P. Ballester, et al. WIREs Computational and Molecular Science, 2020, doi.org/10.1002/wcms.1465