The future of artificial intelligence (AI) is both a matter of uncertainty and a hot topic of debate among the world’s visionaries in technology. The pursuit of AI has been projected as an impending threat to humanity on one side of the debate and as a beneficial extension of our existence on the other.
Despite the continuing controversy, rapid developments in the field continue to unfold, and scientists are intent on mimicking the profound complexity of the human brain in order to teach machines to think like human beings.
Artificial neural networks are devices that mimic neurons and synapses, and memristors are a promising technology for actualizing this goal. In contrast to volatile memory such as random access memory (RAM), memristors “remember” their resistance when a device is turned off and even retain unsaved data in this scenario, making them reliable and stable alternatives.
A new type of neural network based on memristors could help predict future outcomes based on the present — including words before they are uttered during a conversation.
J. Joshua Yang, a professor of electrical engineering at the University of Massachusetts, and his research group have developed a reservoir computing system (RC) that requires much less training time compared to a typical neural network.
RC systems can provide the neural network with the ability to remember while skipping much of the expensive training process — this involves feeding the network with a large set of questions and answers in a process called “supervised learning.” RC systems excel at handling time-varying data such as a stream of data or words, or any function that depends on past results.
According to Yang, hardware-based RCs designed in the past mainly use large optical components. Their system, by contrast, relies on memristors, “which have a much smaller footprint and can be easily integrated into contemporary silicon-based electronics.”
To test their entirely memristor-based system, the researchers split images of handwritten digits into rows of pixels and fed them into the network in the form of voltage signals. The reservoir achieved an accuracy of 83% and required only 110 nodes, which is a drastic decrease from the thousands of nodes required in conventional networks.
Yang’s future plans involve attempting to couple the reservoir system with another type of neural network called a “convolutional neural network” to perform video and speech recognition in real time.
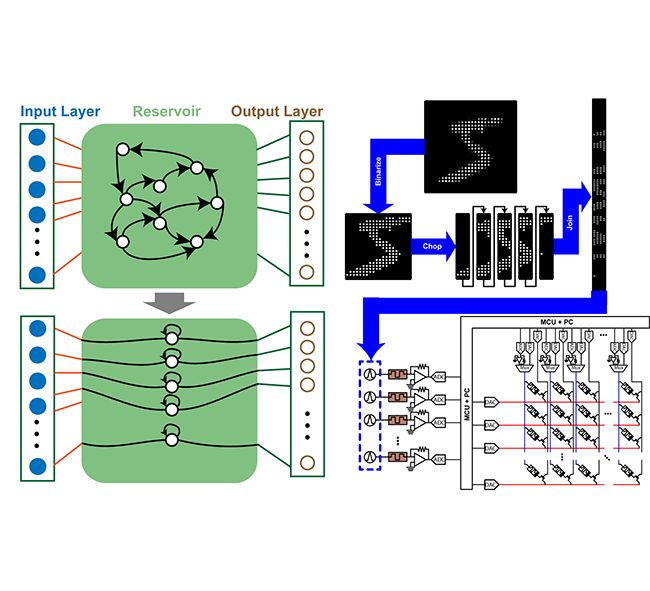