The largest percentage of marine litter consists of plastic waste, in which the biodegradation process can take decades to complete. With serious concerns related to the negative effects of plastic, especially microplastics, on fragile marine environments, a means of identifying, isolating, and extracting these environmental hazards is sorely needed.
The term “microplastics” refers to plastic material with a diameter smaller than 5 mm, commonly generated from the breakup of larger items or otherwise mass produced to match industrial and market needs — think microbeads as exfoliating agents in cosmetics and soaps. What is perhaps even more alarming is the fact that they are found in high abundance at the sea surface, in the water column, and on the seabed, which includes the deep sea. Recent studies have also detected microplastics in freshwater and drinking water sources, causing great concern about their potential threat to human health.
Typical procedures for microplastic identification in environmental samples usually rely on visual sorting by expert users under an optical microscope, although this is only possible when the particle size ranges between 1 and 5 mm. User error notwithstanding, anything smaller than this range goes unaccounted for. It therefore goes without saying that an automated and robust identification and counting method is badly needed to perform effective ecological risk assessments.
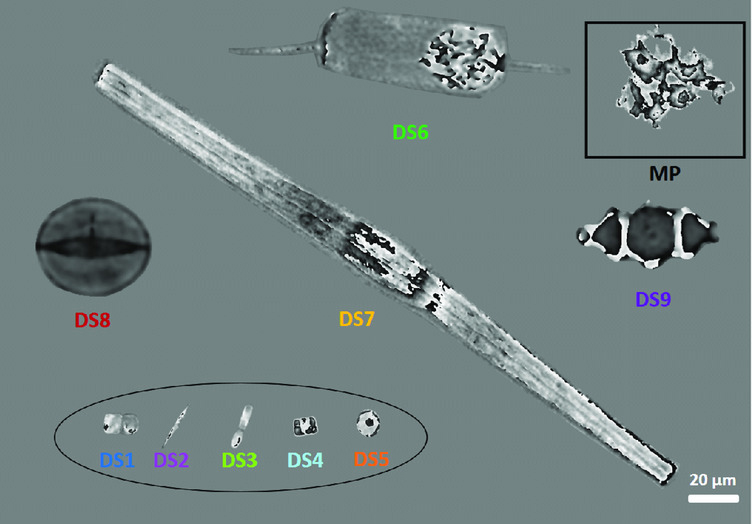
Now, researchers from the Institute of Applied Sciences and Intelligent Systems (ISASI) and the National Research Council (CNR) of Italy aim to solve this problem using a newly developed approach, termed “holographic plastics identification” (HPI), which combines 3D coherent imaging with machine learning to detect microscale microplastics in filtered water samples. Their study was recently published in Advanced Intelligent Systems.
“Screening is highly required to identify plastic material from the other components of a pre-treated water sample during field sampling campaigns,” says Professor Pietro Ferraro, research director at CNR Institute of Applied Sciences & Intelligent Systems. “Unfortunately, the sole method currently adopted to assess the presence of microplastic items inside a liquid sample is a very cumbersome and time-consuming process made by unaided eyes at the optical microscope. Moreover, such analysis is low-throughput and very subjective, since it depends strictly from the operator experience and skills.
“Our research is aimed at providing and automatic and fast screening tool that can identify micro-plastics in water samples over a wide range of scales, from 10 µm to 1 mm size,” added Ferraro. “Within this range, diatoms, i.e. marine microalgae are abundant objects that could be found inside the water samples under test. Hence, the main challenge of this work was to discriminate between microplastics and the very heterogeneous class of marine microalgae.”
In holography, photographic recordings are captured using the interference pattern between two or more beams of light. The holograms that are generated as a result can appear three-dimensional. In the past, digital holography has been used to image microplastic particles, providing a fast and effective means of identification with the potential to provide a low-cost, field-portable system for real-time environmental monitoring. The team plans to take it one step further by combining the technique with artificial intelligence to improve its accuracy and capability.
“We use a holographic microscope to inspect the liquid samples (i.e., marine water),” said Ferarro. “This microscope provides us an image of each sample. Thanks to the tridimensional (3D) imaging capability of the Digital Holographic microscope, we can have at the same time a full 3D view of all the liquid volume sample, thus imaging all solid particles dispersed in the liquid in good optical focus.
“Compared to conventional optical microscopes, the information’s content of holograms is quantitative,” he adds. “We use this large information throughput to extract a set of distinctive features for each object. Some of them are morphological, some other are specifically tailored to describe the microplastic class (holographic descriptors). Essentially, we can say that inside a digital hologram a unique signature of each microparticle is stored. Such signature is due to the interaction of laser light with each of them and we were able to extract such signature.
“This permitted us to identify each microparticle and distinguish between diatoms and plastics pieces. Artificial Intelligence aided in sorting the tow classes. As first step, we examined a large amount of microplastics (thousands of them) and we feed an artificial intelligence with the features measured from them. Then, we repeated the process for various classes of microalgae.
“Thanks to this training stage, the machine learned how to discriminate microplastics from microplankton by looking at this set of features. In the final validation stage, we tested the machine with new unknown objects. As expected, this was able to associate each object to its own class. Experimental test and related results show the successful automatic screening of microplastics into heterogeneous mixtures of objects in water samples, reaching accuracy higher than 99% in classifying thousands of items. We demonstrate efficient classification of microplastics from diatoms in the presence of a wide range of dimensions and a large heterogeneity of sizes, shapes, light absorption properties, and plastic materials involved.”
Real-time, automatic recognition of microplastics in marine water samples is an ambitious goal given that they contain a number of other components, including microscale organisms such as plankton and nekton, that can be easily confused with microplastics. To circumvent this problem, the researchers created a library of holographic images for ten populations of micro‐objects: nine diatom species — a type of single-celled plankton — and a heterogeneous mix of microplastics between 20 μm and 1 mm, which includes polystyrene, polyethylene, polypropylene, polyvinylchloride (PVC), and PE terephthalate.
“After recording digital holograms of the samples, we applied holographic object detection and automatic refocusing to reconstruct the complex wavefronts of each object in the field of view. Then, we extract the modulus‐2π or ‘wrapped’ phase images, from which we segment the detected objects … From the acquired sequences of reconstructed holograms, we identified a total of 2000 objects evenly distributed among the ten classes.”
This methodology was able to recognize microplastics in pre-filtered water samples with 99% accuracy, discriminating them from diatoms and differentiating between different sizes, shapes, and plastic types.
The proposed method could be the first step to automatize the MP identification procedure and make it reliable by significantly augmenting the number of objects analyzed. The aim of this work is to provide an automatic prescreening tool that could replace unaided microscope observation of pretreated water samples.
“The joint action of Holographic Microscopy and Artificial Intelligence could open the route for the development of a fast, high-throughput, accurate and not subjective recognition/counting tool … for in situ monitoring in a near feature,” says Ferraro. “Holographic technology is mature enough and provides new, compact, cost-effective and field portable microscopes. Thus, this work could trace the route for the development of sensor networks to map the abundance of microplastics in marine water and the distribution of their flows.
“This is the first mandatory step to counteract the spread of microplastic pollution in our seas. [Such a] novel Intelligent Microscope can be of uncountable aid to perform accurate wide investigations aimed at evaluating where microplastics are, how many microplastics can be counted, and furnishing their size and shape. Such investigations are almost impossible at the current state of the art due the long times needed so far for inspecting water samples.”
Updated Feb 4, 2020 to include quotes from Pietro Ferraro