3D printing technologies are rapidly expanding to more and more industries, including medicine, materials, food, aerospace technologies and many others, making manufacturing faster, cheaper, and more reliable.
However, with this production method, there is often a shortage or excess of material after printing. If not enough is used, the printed object may be too weak, while too high a material flow rate results in imperfections in the printed samples, and wastes money on production.
To address this issue and make 3D printing more efficient, a research team led by Woo Soo Kim of Simon Fraser University in British Columbia, Canada have augmented the 3D printing process with in situ control and correction of material consumption.
The scientists worked with fused deposition modeling, a 3D printing technique that prints samples layer by layer, depositing melted material in the form of filaments in a predetermined manner where the quality of the printed object depends on the flow rate of the material coming out of a special nozzle. The optimum rate, however, may be different at various stages of the printing process, and the wrong choice of rate can lead to the aforementioned problems
To automatically control and regulate the material flow rate when printing individual components — which in the present study was a plastic dog bone — the researchers turned to machine learning. Their approach uses data analysis to control the manufacturing process to learn from input data, identify patterns, and make predictions.
The camera that was fixed on the 3D printer recorded the printing process, and the data was then sent to the computer for analysis. The machine learning algorithm trained to determine whether the correct amount of material was being extruded at a given moment by the appearance of the part was able to correct the process in real time if the plastic flow rate was deemed to not be optimal.
All this works well on paper, but the scientists needed to test the effectiveness of their algorithm experimentally. To do so, the team printed samples at various flow rates and found that for initial extrusion rates of 60%, 80%, 100%, and 120% of optimal, the material flow rate approached the optimal by the end of the printing process.
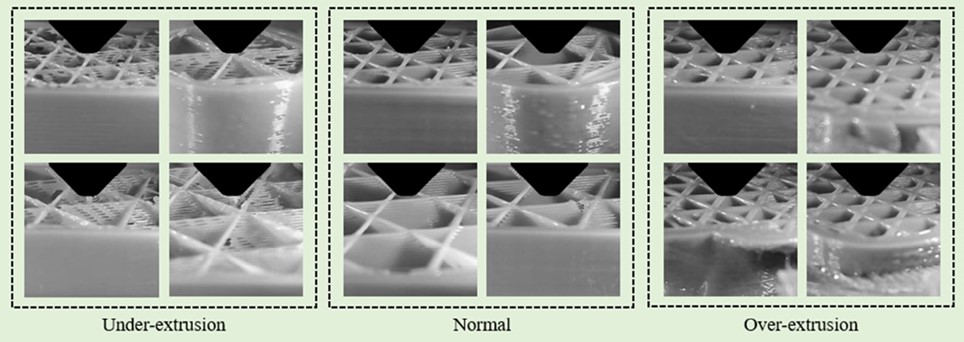
As for the parameters of the printed sample, the results were truly impressive: Compared to printing without using the control algorithm, the new approach resulted in increased sample strength by up to 200%, which may be crucial in many mechanisms, and saved up to 40% of the amount of material.
Despite the significant progress, the scientists believe that further improvement of their approach is possible.
First, they believe that their method can be used not only in fused deposition modeling, but also with other 3D printing methods. In addition, not only could the material flow rate can be controlled in real time, but other parameters of the printing process, such as temperature of the material. Even the printing pattern could be improved by increasing the size of the data set on which the program is trained, and by making print quality checks more frequent.
As always in applied science, only further research and their industrial implementation will show whether these predictions are correct or not.
Reference: Woo Soo Kim, et al., Adaptive 3D Printing for In Situ Adjustment of Mechanical Properties, Advanced Intelligent Systems (2022). DOI:10.1002/aisy.202200229
Image credit: Minku Kang on Unsplash