Imagine there is a flower in front of you: you could see, touch, and smell it. As a human, you have more than one ‘sensor’ to map this flower in your mind. But what if you could only see or only smell? What if you had access to just one of the many dimensions that collectively make up the physical concept of a flower?
Conventional sensing systems currently do not have the same sensing capacity of living creatures; they usually possess only a single sensor (or sensor array) with which to observe an object of interest. When considering the increasing demands on high-performance systems, any single sensor has its limits in terms of sensory precision, accuracy, and sensitivity.
One solution to this problem involves increasing the number of sensor types to form a multi-sensor system.
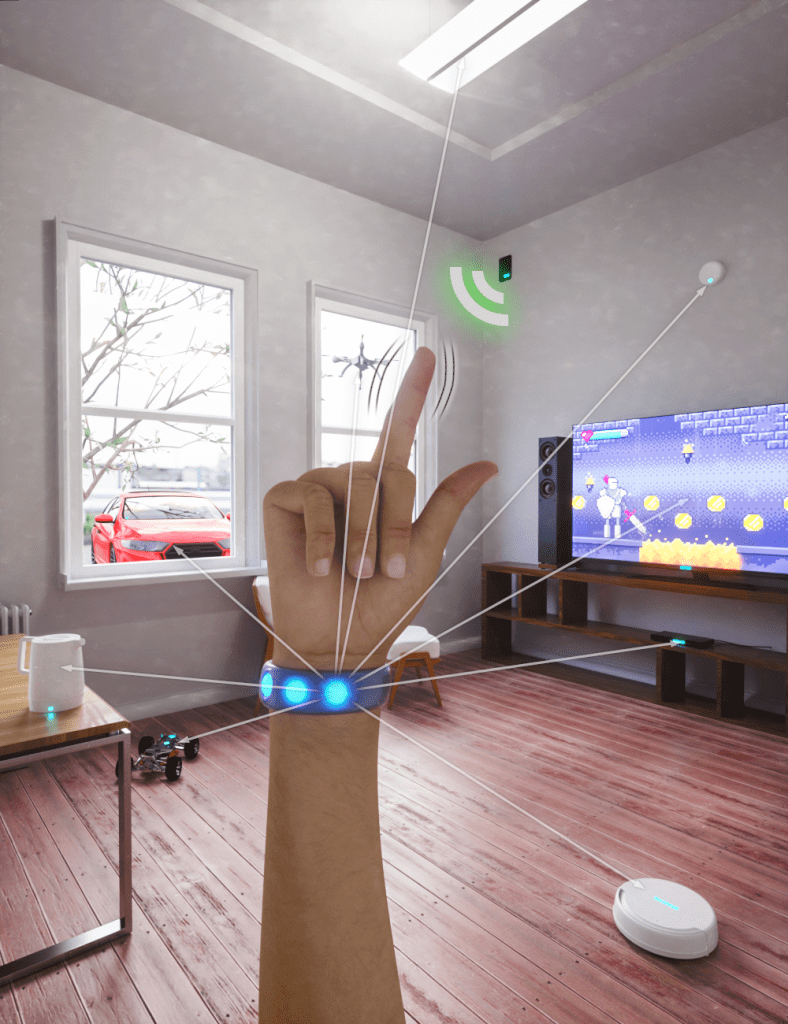
The system fuses data from radar and pressure sensors to recognize hand gestures with over 90% accuracy.
Multi-sensor systems can provide more than one method to investigate an object, such as a flower, which helps to more accurately capture the object’s condition.
Physically connecting various sensors into a single intelligent system is not complicated. However, the main challenge is the organizational relationship between them, which must be optimized in order to fully exploit the heterogeneous information being delivered. Each type of sensor has its own strengths and weaknesses and, in the sampling process, different sensors tend to return data types which differ in terms of sampling rate, data format, and the physical information behind the data.
We need a mechanism to deal with these differences, to combine the sensors’ strengths while suppressing their weaknesses. For example, when we have two different sensors in a decision-making system, and the system is returning opposing (positive and negative) results, to which information should we listen? To what should we give more weight?
In a study published in Advanced Intelligent Systems, researchers from the James Watt School of Engineering at the University of Glasgow propose a multi-sensor data fusion mechanism using an hierarchical support vector machine (HSVM) algorithm to effectively fuse different data types.
HSVM consists of multiple layers of modified SVM classifiers; the results and confidence levels of each layer are delivered to the following layer, fusing the data coming from the different sensors (see video to learn more).
This concept was validated by combining contactless (radar) and flexible, wearable (pressure) sensors into a novel gesture-recognition system, with results gathered from 15 different participants. It was shown that both sensors were able to independently achieve gesture recognition, but by fusing the data from the pressure sensors (which monitored the subtle movements of tendons around the wrist), and the contactless radar sensor (which captured the dynamics of finger movements), the accuracy of gesture recognition was improved significantly. Alone, the pressure sensors and radar were about 69% and 77% accurate, respectively. However, using the new fusion concept, the accuracy of the multi-sensor system reached over 90%.
A further difficulty for the smart organizer in this test system is that for any natural sequence of human gestures, the pressure sensor data will be meaningful in a static state (fingers kept still), whereas radar data will be more meaningful in a dynamic state (transition between static states as captured by the Doppler effect).
These natural differences lead to the incompatibility of simultaneously received data, a challenge to fusing the features extracted from both sensors at any given time. Compared with other sensor fusion algorithms, like soft voting, HSVM exhibits an excellent advantage in this difficult situation, since useful information can be extracted in different layers.
With these prominent advantages, HSVM could be a promising candidate for the organization of data from complex multi-sensor systems.