For the couples involved, in vitro fertilization is an expensive, invasive and exhausting procedure. The success rate depends on a range of factors, but for women under 35 it is only 40%. Any technology that might improve this rate at all would be a welcome addition to the field.
One particular form of in vitro fertilization called “intracytoplasmic sperm injection” involves the direct injection of sperm into the egg. At present, sperm are selected for this process based on their appearance. However, the literature contains conflicting accounts as to whether there is any correlation between the appearance of sperm and its fertility. The studies that do claim a correlation do not agree on the physical aspect of sperm that is linked most strongly to fertility. This makes the choice of sperm for fertilization an exercise in educated guesswork.
A team from Canada recently sought to use a machine learning algorithm to better predict the fertility of individual sperm based on their appearance.
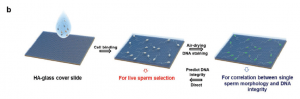
The imaging process.
They developed a test in which individual sperm became chemically bound to a transparent plate, which allowed for rapid assessment of five different aspects of sperm appearance. At the same time, an assay was run to assess the integrity of the DNA in the sperm (this process is shown above).
Using DNA integrity as a proxy for fertility, the team fed the data into a machine learning algorithm. Interestingly, this process showed that while there were correlations between individual characteristics and fertility, these correlations were not strong.
By simultaneously assessing five different physical characteristics, the algorithm was able to accurately predict DNA integrity 85% of the time.
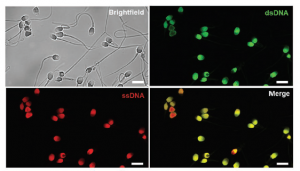
The imaged sperm.
The process of binding sperm to the glass plate can be used in conjunction with in vitro fertilization. This means that it would be possible for sperm assessed using this technique to be directly used in the clinic. However, before this stage the team hopes to acquire data about a variety of other sperm characteristics such as “tail beating profile” in order to further improve the success rate of the algorithm.