Image credit: Shutterstock
With the rapid advancement of artificial intelligence (AI) and machine learning technologies, the demand for computing power is ever increasing. This becomes even more important as Moore’s law reaches its limit in which the number of transistors on the most complex chips reaches a maximum. Improving chip performance and energy efficiency for data-centric applications becomes more and more difficult with current technologies.
To this end, scientists are enthusiastically looking for new computing devices and architectures to meet the needs of data-driven computing. Neuromorphic computing, inspired by the brain, was proposed in the 1980s and integrates tiny functional electronics circuits in a way that mimics the biological architecture of the brain. In comparison to the conventional von-Neumann computing architecture (used in most computers today), brain-inspired computing architecture are highly energy efficient and have superior computing abilities.
The brain is a highly complex, nonlinear, and self-organized system that efficiently uses complex dynamics to perform its “computations”. The only way to truly mimic its vast functionality is to better emulate its hardware. For this reason, artificial neurons built with active memristors— which are non-linear memory devices with programmable resistance— have become one of the most promising candidates to build bio-realistic neuromorphic systems.
As mimics for biological neurons, which transmit information through complex electrical signals, memristive neurons have already achieved most of the known biological neuron dynamics, such as all-or-nothing spiking of an action potential, bursting, and a refractory period, among others. A circuit composed of only two active memories and four or five resistors and capacitors components can realize the biological neuron dynamics that can be achieved by using thousands of transistors in the conventional semiconductor circuit. This greatly reduces the number of devices and power consumption. However, it is worth pondering how to apply these dynamics for computation.
In a recent study published in Advanced Intelligent Systems, Professor Xinjun Liu from Tianjin University and co-workers demonstrated the implementation of bio-realistic bursting dynamics in artificial neural networks. Bursting is a well-known dynamic feature of biological neurons. In general, a burst is composed of two or more spikes followed by an inactive period. Neurons can fire single spikes or bursts of spikes, depending on the nature of stimulation and the intrinsic neuronal properties. Memristive neurons can generate different numbers of spikes in a bursting cycle when they are excited externally. A simple pattern classification function is realized by encoding the number of spikes.
Sequential computing in the traditional von Neumann architecture leads to low energy efficiency when dealing with problems such as pattern recognition. In comparison, the human brain is very efficient in processing these tasks due to its parallel computing capabilities. The artificial neural network composed of memristive neurons has the characteristics of low power consumption and parallel computing, both integrated into neural networks, where the bursting dynamics are used to encode and transmit information.
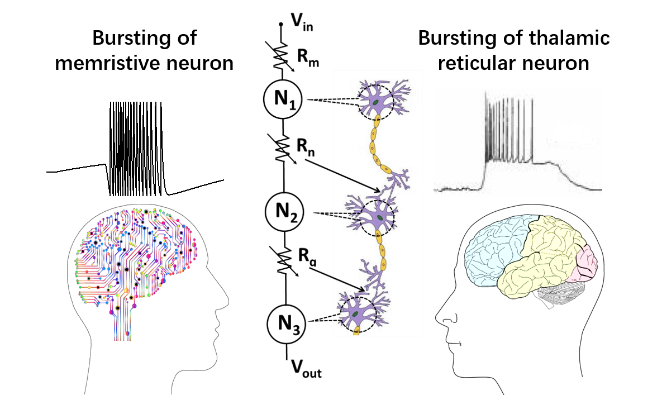
Importantly, bursts have multiple spikes that are used to activate the next synapse, which means that the stability of information during transmission is greatly improved. Bursting dynamics encoding information endows the artificial neural network with biological significance in computing. Linking memristor neurons, biological neuron bursting dynamics, and artificial neural networks together is a breakthrough discovery. As such, it will go a long way to better understand the working mechanism of biological neuron dynamics and memristor neural networks that mimics biological neuron networks.
“It is very important to find the connection between the biological neurons and the memristive neurons,” says the study’s first author, Yeheng Bo. “This is an important step for humans to understand how the brain works”. Simulating the dynamics of biological neuron directly with the hardware can potentially make the computing energy efficiency close to the real human brain.
Reference: Yeheng Bo, et al. ‘NbO2 Memristive Neurons for Burst‐Based Perceptron‘ Advanced Intelligent Systems (2020). DOI: 10.1002/aisy.202000066