Nowadays, artificial intelligence (AI) and computer programs have infiltrated almost every corner of our lives; from facial recognition, language translation, image and video production, to self-driving cars and personal care aides. Other applications that might not yet be mainstream knowledge have to do with scientific exploration and research and development. For example, AI has the potential to revolutionize medical practices through augmenting medical diagnosis and have found application in drug discovery. In many places, researchers have also attempted to use AI algorithms to manipulate biology, chemistry, and physics with different setup configurations that can detect DNA modifications caused during epigenetic regulation or gene mutation, choose the most optimal reaction pathways in synthesis, and search for exotic particles using adapted learning networks.
Now, AI is extending its reach to the chemistry lab, beyond just simple reaction planning. In traditional chemical labs, reactions are carried out by experimenters in individual beakers and conical flasks, where substance transfers, experiment setup, reaction monitoring, and product purification are all carried out by hand.
Replacing these time-intensive practices with machines and computer programs is directing the future of modern synthetic chemistry, where human labor is no longer needed, experimenter variability is reduced, and the barrier for technology translation and expansion is lowered. A future scenario might require a new chemistry student to receive minimal technical training; they could hypothetically remotely perform parallel experiments in a laboratory while relaxing in a café or bar with a drink in one hand and their iPad in the other. Moreover, when risk factors are involved, such as reactions using strong acids or explosive components, unmanned systems are always favored.
Though partial automation has been adopted in industrial chemical plants, this level of automation is nowhere near comparable to that of car-making manufacturing plants, where the complicated assembly of large vehicles proceeds efficiently in an almost human-free environment.
The idea to fully automate chemistry and chemical engineering at the lab scale, in which AI independently explores new reactions and optimizes conditions, is still in its infancy. However, several scientific teams have made strides towards its realization.
A notable example is Chemputer, which was developed by Professor Lee Cronin and his team at Glasgow University, and promises to revolutionize drug discovery. The chemical-robot system (hence its name) functions much like a bench chemist would: it automates the synthesis of pharmaceutical compounds by uploading blueprints of the chemical procedures into machine-readable steps, which are sequentially conducted by robots with embedded sensors to judge the accomplishment level of each step before proceeding to the next. Meanwhile, scientists at MIT have designed a platform of algorithms that plan effective schemes for synthetic chemistry by “learning” from millions of published chemical reactions, and then command robots to carry out the task.
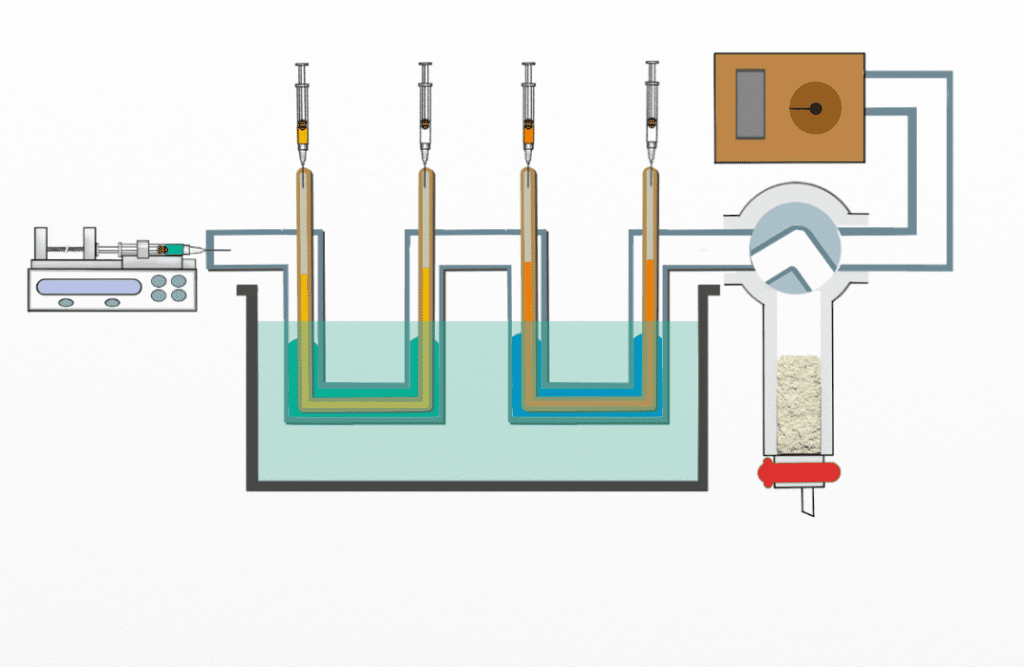
Another strategy to automate synthetic chemistry involves microfluidic systems, which first emerged as an analytical tool in 1992 and offer the capabilities of entire labs in a miniaturized format. Microfluidic systems transport, mix, and separate liquids in a streamlined process. This technology has been gaining popularity not only in chemistry, but also in biology, materials, and medicine, as experiments can be run in a precise, time-efficient and cost-effective manner. They adopt modular designs, with each module performing a particular task, such as reagent mixing or product purification—in this way, multiple reactions can be run in parallel or sequentially, without the need to interrupt the reaction at each step.
Professor Shaohua Ma and his team at Tsinghua University have used a microfluidics system to perform synthetic reactions which are challenging or impossible using traditional techniques. On the bend, the reaction proceeds in multiple steps, with each step having multiple (unwanted) possible outcomes or “tracks”, which are difficult to control. To overcome this challenge, the authors developed a microfluidics system composed of several modules arranged in a sequence, with each module performing a specific reaction task. Each event in the reaction sequence is controlled using a computer program that uses syringe pumps that feed liquid into the reaction through rubber tubes.
An advantage to this design is the ability to control reactant quantities during a reaction through molecular diffusion-controlled mass transport. Smaller molecules transport across the porous channel wall (membrane) at higher rates than the larger ones. The relative quantities can be tailored by using membranes with different pore sizes. When one reactant with multiple reactive sites substantially overwhelms another, the reaction that occurs by coupling two partners becomes mono-selective and proceeds toward one specific direction. Without the extreme ratio established using the porous membrane and microfluidic control, the reactant would react with multiple partners and the reaction becomes non-selective again.
A standard microfluidic system — excluding the syringe pumps that are standard instrumentation in fluidic chemistry — cost tens of pennies for the consumable plastic tubes and is relatively easy to be assemble compared to highly technical chemistry that is sometimes performed in the lab. The computational load is rather low as the command controls (algorithms) are programmed linearly following the reaction logistics; the program only runs forward with temporal pauses and initiates, but no backward actions that form loops. When the computational cost is reduced, the software does not require a working station, but can be run using a programmed app on a cell phone.
It’s interesting to imagine a world in which research chemistry is done through such modular systems. The modules could be standardized and molecules more readily manufactured on large scale, with expenses reduced and quality guaranteed. Each system, including its commanding program, is highly customizable and dependent on what the user needs, allowing for easy reproducibility as the exact same system can be set up in labs around the world. It would make exploratory chemical experimentation much simpler—essentially reduced to options on the screen—and would eliminate the need for lengthy design-synthesize-test iterations.
However, before the concept of “intelligent chemistry” becomes a reality, there are still many challenges to overcome. Real-time chemistry is far more complicated than a simple set of reaction choices. This area of research, as with many others, requires an even higher degree of creativity and ingenuity to solve than AlphaGo, and perhaps AI is not yet at this level.
In addition, when an automated system is developed to create unmanned solutions for a specific reaction type, it might not be suitable for another, just like an algorithm developed for autopilot cannot be directly translated to diagnose a tumor, even when both are derived from computer vision technology. Even for one target reaction, for the algorithm to perform on a level that would allow it to surpass human performance, this still requires iterative optimizations and increased computation loads. This makes improvements to computational capacity and reducing the hardware size even more challenging.
Regardless of the challenges, we are expecting the next generation of AI that is nurtured by the growth of new computational methods, such as the optoelectronic computation that may break Moore’s law, and new neural architectures. The future of AI is expected to perform tasks far exceeding the capability of the current technologies, promising to conquer a myriad of challenges. And we believe this will happen sooner rather than later.
Research article found at: X. Pang, et al. Advanced Intelligent Systems, 2020, doi.org/10.1002/aisy.201900191