Artificial intelligence enabled MOF inverse design. Graphic courtesy of Dr. Zhenpeng Yao, University of Toronto
Natural gas and biogas are a potential source of methane (CH4) and CO2 for feedstock chemicals, providing they can be separated. The CH4 component can serve as a feedstock for chemicals, a fuel and for heating homes, buildings, and driving thermally powered industrial processes, while CO2 can be used as a feedstock for making renewable chemicals and fuels. Taken together, CH4 and CO2 — two potent greenhouses gases — can be converted into a renewable chemical feedstock that can be used to generate green commodity chemicals, such as hydrogen and carbon monoxide, both of which enable a sustainable economy (see for example www.solistra.ca).
Porous materials with surface structures that are based upon periodic arrays of nanoscale voids are renowned for gas separation based upon the size, shape, and adsorption energy of the constituent molecules — the most celebrated of which historically are zeolites and molecular sieves. The subsequent discovery that nano porous solids can pervade the periodic table of the elements has greatly expanded the opportunities for improving gas separation performance indicators, examples being aluminophosphate ALPO, silicoaluminophosphate, SAPO, metal aluminophosphate MeAPO, and metal silicoaluminophosphate molecular sieves, as well as metal organic framework (MOF), covalent organic framework (COF), and zinc imidazolate framework (ZIF) classes of porous materials.
To search for champion gas separation materials experimentally and computationally, the main properties of interest include surface area and pore size, adsorption energy, and capacity. MOFs are exceptional in this regard because of the essentially infinite structure-composition space they portend and hence the colossal possibilities they offer for tuning the properties that underpin the efficient uptake and separation of gases such as CH4 and CO2.
In this context, a recent report described an inverse machine learning strategy for the accelerated discovery of high-performance MOFs that can affect this important gas separation process. There has been a lot of generative model research on molecules, and many on solids. MOFs are “somewhere in between the two”, which bridge molecules and crystalline solids and add tons of complexity.
This strategy is akin to the autonomous design of molecules in organic chemistry, which involves the encoding process: dimension reducing and converting discrete molecules into continuous vectors using neural networks followed by the decoding process. Continuous representations allow the automatic generation of novel molecules by sampling the latent space. More importantly, they also make the use of powerful gradient-based optimization feasible to efficiently guide the search for optimized functional molecules.
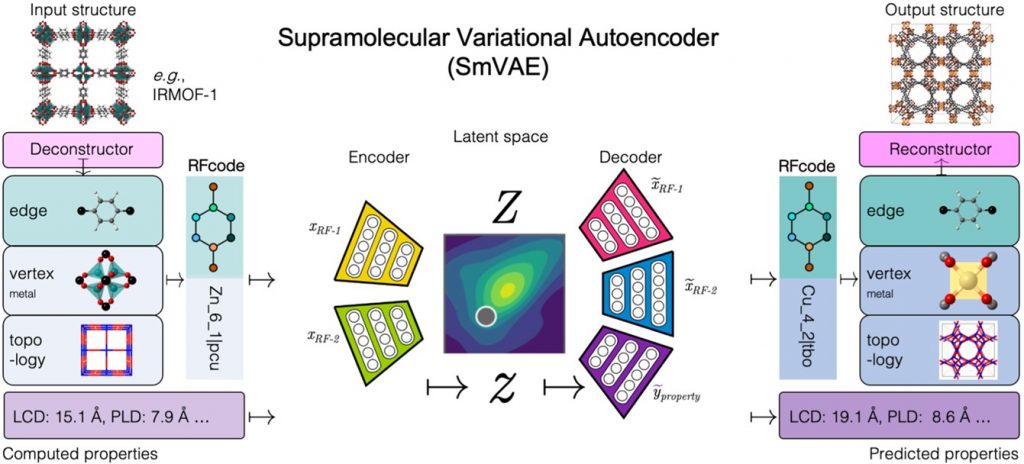
Starting with the invention of a concise yet effective representation for MOFs, a similar tactic is being adopted in computational materials searches whereby instead of selecting building blocks/precursors to create a material with targeted properties to achieve a desired functionality, the process is being run in reverse so that a desired functionality is stipulated and the building blocks/precursors to create the properties to generate the functionality are sought.
In a demonstration of the inverse design approach of frameworks, the function of CH4-CO2 gas separation was picked. By defining the targeted property-function relationship in order to optimize adsorption energy, capacity, and selectivity of the CH4-CO2 separation, the number of possible candidates that offer the desired performance metric will be significantly reduced compared to the direct approach, thereby enhancing the chances of realizing the material synthetically.
Among the top candidates identified, the champion MOF that emerged from this study displayed an CO2 adsorption capacity of 7.55 mol/kg at 5 bars, 300K and a low concentration of CO2, with a preference of 16 compared to CH4, performance that matched or bettered prior art nano porous CH4-CO2 separation materials.
The test of the reliability of this massive inverse design search will be whether the materials are synthesizable, and the whether predictions are born out experimentally by humans and/or machines.
The takeaway from this study is going backwards in materials discovery through an inverse design strategy can mean going forwards in materials science and engineering towards a practical technology.
Reference: Yao, Z., et al., Inverse Design of Nanoporous Crystalline Reticular Materials with Deep Generative Models, Nature Machine Intelligence (2021) DOI: 10.1038/s42256-020-00271-1