Image credit: Gertrūda Valasevičiūtė on Unsplash
This opinion editorial was inspired by a simple question: Where have all the equations gone in a post-theory materials chemistry world?
The field of chemistry is undergoing a rebirth and is morphing from a hands-on practice into what many are now calling “algorithmic chemistry” — a mysterious world powered by data and statistics, enabled by the algorithms of computer science, and touted as the brain power underpinning the accelerated discovery of all forms of matter in what some conjecture a post-physics world.
But what does this mean for the future of the field?
How does a materials chemist think?
When learning materials chemistry, solid-state physics is key. However, many materials chemists, especially those more focused on making materials, succeed in the art of synthesis more by the creative juxtaposition of the elements of the periodic table, trends in their properties, and generalization of the concepts and principles rather than a deep-seated understanding of how to work out the mathematics and physics underpinning the solid state.
Materials courses are interdisciplinary, and for those who have come from outside of the chemistry department, the goal is not to learn all the theories and the math behind it, but rather to learn how a materials chemist thinks and functions. This point is stressed to emphasize the challenges of working collaboratively, where understanding and benefiting from each other’s strengths is the secret to collective success.
Even when one does not use mathematics to understand all the axioms, postulates, and laws of physics to solve all the relevant equations in condensed matter physics, one can still appreciate and make use of the information within those laws to make materials. For example, if one were to try to develop a room-temperature superconductor — a resistance free material that would revolutionize power transmission, energy technology, rail transport, and computation — a materials chemist would first understand and relate the physical laws and theories that determine a material’s properties (many of which are often interrelated), and then try to design a synthesis that builds all these ideas together.
One does not need to prove from first principles every mathematical step in an equation to go into the laboratory. Instead, one needs to identify and understand the meaning and implications of pivotal terms in master equations that emerge from these theories. They provide the principles, tucked inside recognizable mathematical and physics equations, that help scientists experimentally realize target materials with desired property, function, and utility.
AI’s missing link in chemistry
This is where there may be a challenge for materials chemists that needs to be addressed with the introduction of artificial intelligence (AI) and machine learning in research. This is because the computational methods and algorithms used are often devoid of the language of mathematics and physics that we recognize as connected to the field of chemistry.
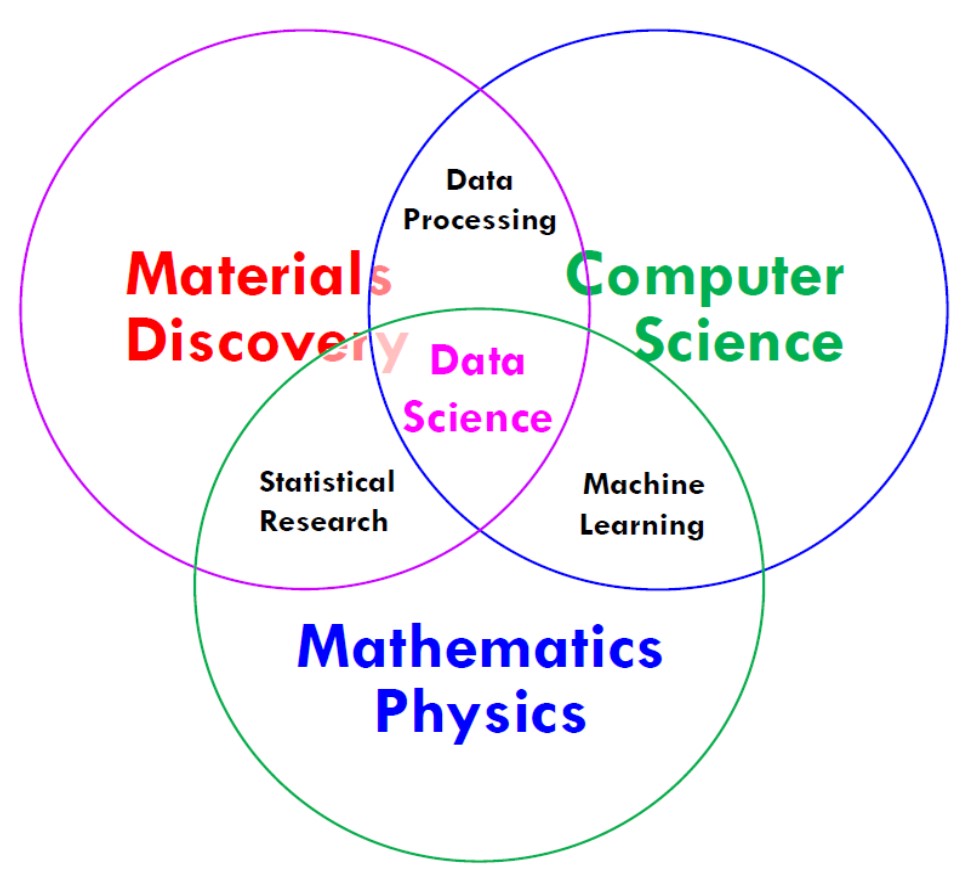
Do not misunderstand our intentions, we are very enthusiastic about AI and machine learning in research. We are not saying there are no mathematical equations in the algorithms that power different classes of machine learning. However, amid all the coding that underpins algorithms, couched in the language of computer science and making better and better predictions as they are fed with more and more data, there seems to be something missing for the materials chemist.
While these equations can successfully make predictions by finding patterns in or numerically optimizing through large amounts of data, the models they produce do not seem to convey any physically meaningful information provided by the typical equations that one can find in the natural and physical sciences. For example, each term and symbol in Schrodinger’s and Maxwell’s equations has its own meaning. On the other hand, the outputs of a machine learning search — for example, a well-optimized material within a fixed set of criteria — may be excellent for achieving certain goals, but they do not tell us the reasons why one may obtain that particular material.
A potential problem with this could be the risks of drawing incorrect conclusions from the outputs of AI since, depending on how a certain algorithm is structured, it is possible for different sets of data — potentially with completely different meanings — to produce the same prediction.
Using data to extract patterns and predictions seems to be part of how computer, data, and automation scientists think. There is nothing wrong with that, and it has been incredibly successful for applications such as image and speech recognition. However, in our opinion, it is not how most practitioners of chemistry are trained to think and experimentally synthesize their ideas in the laboratory — we are trained to work with tried-and-true concepts and principles from which we can make connections and inferences.
If the outputs of machine learning only allow us to extract patterns or successfully make a certain prediction, in many cases it may still be up to us humans to provide meaning to such models to completely understand and solve a problem. A case in point is AlphaFold, which did an excellent job at predicting protein structures, yet many still believe still has not revealed the inner workings behind protein folding for the “protein folding problem” to be considered solved. One can also imagine a similar problem for making predictions and understanding the structure-property relationships of so-called “strongly-correlated” materials and many other examples in the solid state.
Many chemists may feel deeply uncomfortable with materials discovery free of the theories with which they have been trained. Instead, many now are faced with a tsunami of AI and machine learning algorithms designed to accelerate the rate of materials discovery, which to most chemists is comprised of unintelligible, nested computer code. This problem is known as “explainable AI”.
The interface between chemistry and AI
The challenge with AI is that it transcends physics. Dubbed the “fourth paradigm” in science, an all-encompassing theory in the understandable language of physics does not yet exist. This high level of computer complexity and the inability to understand AI in a familiar language is currently presenting a barrier to most chemistry students, researchers, and teachers interested in benefiting from it in materials discovery.
Some may say that this should not be perceived as a problem if the predictions are correct and can be borne out in practice. However, since the laws of nature and its inner workings can be extremely complex and interconnected, we would like to be more conservative when it comes to this issue. We also suspect that many others outside of mainstream AI and machine learning might wonder: How can materials chemists trust what comes out of a black box if we cannot provide any significant meaning to how it got there?
In chemistry, the quality of a machine learning model can depend on how one applies chemistry to it and the interpretation of the best-fitted parameter is only as good as the quality of the data we feed into it; data whose meaning and information could be a lot more layered and complex than, for example, the overall shape of an object used in image recognition.
However, there is no going back in the quest for accelerated materials discovery in a fast moving, highly competitive world. The arguments for rapid searches of gigantic chemical spaces for identification of a molecule, polymer, or pharmaceutical to reduce time-to-market and cost of a product are compelling — a case in point being vaccines in a pandemic.
With the time-sensitive catastrophe posed by climate change looming, high-speed discovery of energy materials that enable the transition from fossil to renewable energy systems makes sense. We believe chemists must learn to live and work harmoniously with AI and machine learning if we are to remain relevant.
From a chemist’s perspective, we believe that in addition to predicting certain outcomes and optimizing performance, machine learning should also be applied more towards helping us with various aspects of fundamental research (as opposed to purely applied research) in chemistry because there is still so much that we do not understand, and an improved understanding could be the key to many new innovations.
While Pablo Picasso once said, “Computers are useless, they can only give you answers”, in chemistry, the key is to keep on asking the right questions and to understand how we can best work with — yet not blindly rely on — this new technology to help accelerate not just materials discovery but the multi-scaling challenges faced by materials scientists and engineers taking our innovations to the marketplace.
Written by: Geoffrey Ozin1 and Andrew Wang1,2
Solar Fuels Group1 and Matter Lab2, Chemistry Department, University of Toronto, Emails: [email protected], [email protected], Websites: www.solarfuels.utoronto.ca, www.matter.toronto.edu